Recently we've been working on how we could deploy models written in R into a production system, where they could be called as part of a data pipeline or on-demand. From a data engineering point-of-view one of the best tools in your toolbox when you want to execute relatively small pieces of code, without worrying about all the infrastructure or software platforms supporting it, is Azure Functions. Functions also have an extensive range of bindings supporting different input and output sources. As it stands today both R and Python are supported in Azure Functions but only experimentally with a limited number of bindings. There's a pretty good introduction over on Microsoft's Azure blog with supporting code over at Github. This is based on using PowerShell to provide a Timer Triggered function which executes an R script. Unfortunately, PowerShell is also currently experimental in Azure and has a limited number of bindings available to it, but it's a good place to start and gets up and running with R, which requires a site extension to be installed and some manual unzipping (the process isn't currently as smooth as it perhaps could be). This got us up and running and demonstrated that we could run R code through Azure Functions; but we wanted to use other types of bindings and to have something a little more reliable (and testable) than PowerShell. Azure Functions (v1) supports several languages, but the key ones are C# (C# or C# Script) and JavaScript. We ran into some early issues with C# as an option with the R.NET package and target architectures and so moved over to JavaScript to see if we could get something running a bit quicker. Initially we started working with Child Processes but soon came across a package called r-script which takes care of getting data into the R script, handling the response, and provides both synchronous and asynchronous methods. It's one of those neat little packages which just takes the mundane work away from you. One thing it does make available is an R method called "needs" which handles the installation and usage of packages in your script and is the recommended way of doing this using the library. So, what do we need to do to make it work? First we'll need to make sure the environment has all of the tools needed to developer R code and Azure Functions, this includes ensuring that you have NodeJS and NPM installed which I would recommend using a version manager for. Once this is set up and we've created the template for the function we'll need to ensure that r-script is able to locate the R executables in the path, one way of doing this is to ensure that the R_HOME variable is configured in the environment variables by either setting it manually, or better setting it using an application setting for the project. Once that's done then we can define the location of the executables in the code and append this to the path. Note that here I'm presuming a target OS of Windows.

You'll notice that I've also got another environment variable (application setting) defining where the R scripts are being kept. It's worth highlighting at this point that ensuring the use of application settings will help when you deploy to Azure as the R executables will typically be at a location such as "D:\home\R-3.3.3\bin\x64", you may not have that location available locally so using settings will make the code more portable and maintainable. Once that has been configured then we can simply call the R code using the methods provided by r-script and capturing the output. Make sure you have a read of the r-script documentation to see how to pass data in and get it back out.

We can then do any post-processing we need to with the output object and send it on it's way to the output location. In the case of an HTTP trigger this would be returning it back to the caller.
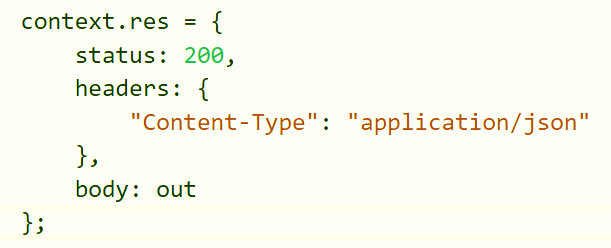
There are some important things that we encountered (and just generally) that it's worth mentioning here. Choosing the right plan for your Functions is crucial, in our investigations we found that running the R scripts when deployed using a Consumption Plan was significantly slower than when compared to it running using an App Service Plan (20+ seconds compared to 2 seconds in our case). Depending on how you want to test or deploy your function this may be fine, but it's something to keep in mind if you're encountering performance issues on a Consumption Plan. Get used to working in the Kudu console, it's incredibly useful for testing your R script and bits of JavaScript as well as viewing the streaming log files. Testing functions locally will save you a great deal of pain and will help debugging your functions.